How to make your data more reusable: success stories
Academic publishers and funding agencies are increasingly encouraging researchers to share research data which underlies their research findings. There is evidence that when researchers use openly available datasets they can build upon existing research and make a greater impact across disciplines. In this blog, we explore the benefits of sharing data openly and share data reuse success stories.
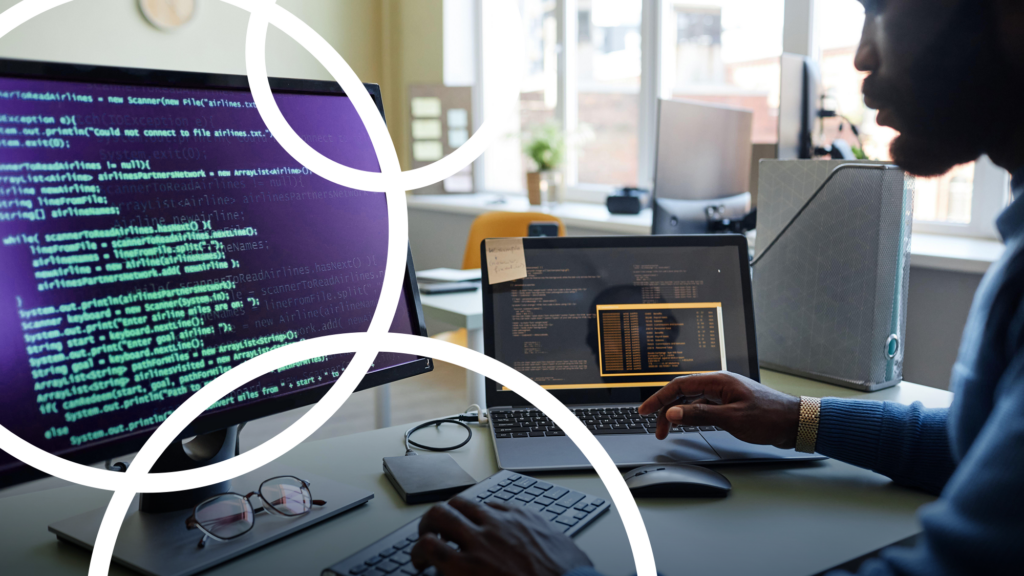
What is open data?
Open data is a crucial component of open science and refers to data that is available for everyone to access, use, and share. It’s often associated with the FAIR Guiding Principles, which aim to make data more Findable, Accessible, Interoperable, and Reusable.
Open data on rise
The open data movement is gaining momentum, with many governments and international organizations across the globe announcing open data initiatives and policies that support data reuse.
For instance, the UK Government has long advocated for open data through data.gov.uk. Officially launched in January 2010, this project has made more than 30,000 non-personal UK government data sets available while supporting data reuse.
Additionally, the EU 2019 Directive on open data and the re-use of public sector information (Open Data Directive) attempts to enhance the way research data resulting from publicly-funded research is made available, accessed, shared, and reused. Following the ‘open by default’ principle, EU member states need to develop national open access policies for publicly-funded research data. Plus, new rules on reusability are applied to such data which is accessible via open repositories.
Plus, in April 2021, the European Union launched the data.europa.eu portal. This open data portal is the point of access for EU member states to public data published by EU institutions, agencies, or other bodies.
More recently, the U.S. National Institutes of Health (NIH) issued their Data Management and Sharing (DMS) policy requiring most grantees funded after 23 January 2023 to create and follow a comprehensive plan for how their research data will be managed and shared. This policy promotes sharing scientific data, accessibility to high-value datasets, and data reuse for future research studies.
How can researchers share their data while facilitating data reuse?
The first step is to identify all research data collected or created as part of a research project and prepare it for sharing. Scientific data can take different forms, including survey results, gene sequences, software, code, algorithms, images, and audio files.
Openly sharing data may not always be feasible due to ethical considerations or other restrictions. This is especially true in fields where the data collected relates to human research participants, such as medicine or the social sciences. Data sets that contain personal data can often be shared openly by obtaining informed consent for data sharing or applying appropriate anonymization techniques and/or controlled access to the data.
After identifying and preparing data for sharing, the next step is to deposit it into a data repository. A repository is a location on the web for data to be stored and accessed by others. Uploading datasets using open, non-proprietary file formats is considered best practice as users don’t need to purchase proprietary software to open them. Additionally, when depositing research data, creators can add contextual information known as ‘metadata’ and receive a persistent identifier (DOI). Persistent identifiers are vital as they remain constant, even if the location of the digital research outputs moves.
Plus, applying an open license is very important as it allows data access and reuse and explains what others can and cannot do with the published data. Researchers worldwide use Creative Commons licenses and public domain tools to share their research and data. Creative Commons open licenses allow researchers to retain their copyright while allowing others to copy, distribute, and use their work. Data sets published under Creative Commons Public Domain Dedication (CC0) or Creative Commons Attribution Only (CC-BY) permit maximum reuse by others with minimum restrictions.
Planning for managing and sharing data can be challenging if left for the end of a project. Creating a detailed data management plan (DMP) before research begins can help ensure efficient data management and make data FAIR. A DMP is a living document that describes how research data will be generated, stored, used, and shared. The document can change and evolve throughout a research project.
What’s the use in reuse?
Benefits for the sharer
Researchers most commonly share their data for reproducibility, allowing others to verify or build on results. Scholars might even openly share data they are not using themselves.
When another researcher uses the data, this contributes to reducing research waste in the field. In addition, other researchers might take the available datasets in innovative and creative directions or use them in ways that the data creator could not do due to a lack of equipment or relevant expertise.
Furthermore, researchers often liaise with the original creators when reusing existing data, asking for additional information on data collection or the conducted study. This can lead to new collaborations, partnerships, or creative initiatives that may only have occurred if the data had been shared openly.
Lastly, when their data is licensed and reused, the creators can receive credit and attribution for producing the original datasets through data citations.
Benefits for the user
Researchers or other stakeholders may use existing datasets within their studies or conduct additional analyses on existing research questions. Such pre-made datasets enable scholars to start working on their research immediately.
Furthermore, in some cases, researchers can access the methodology associated with a research project. For example, specific F1000 article types such as Data Notes allow the full reporting of methods alongside the associated research data.
This way, a researcher knows the results and how they were produced beforehand. Using others’ data helps scholars start their analysis and generate results quickly.
Plus, the reuser does not need to curate or upload data to a repository. When they reach the point of publication, all they need to do is cite the data’s original source using the dataset’s persistent identifier.
Reuse in action
But what does data reuse look like in practice? F1000 publishing venues are home to many research projects that have provided the basis for further scientific discoveries using open data. Take a look below at two data sharing success stories from authors who have published on F1000Research, F1000’s flagship open research publishing venue.
From sugar production to bioethanol production
In 2017, Riaño-Pachón and Mattiello published their Data Note ‘Draft genome sequencing of the sugarcane hybrid SP80-3280‘.
Sugarcane is a key waste product in sugar production and a complicated entity. A polyploid species, it has up to 130 chromosomes with a genome size of 10 gigabase pairs.
Up until the point of the publication of this article, only partial or transcriptome sequences were available. Riaño-Pachón and Mattiello were able to generate the full genome and made it openly available to others. In describing their sugarcane dataset using a Data Note, the authors enabled other researchers to identify and characterize new genes in this crop.
One year later, Santiago et al. used the open data in the Data Note to identify 92 expansin genes in the sugarcane genome in their Research Article.
The leaf of the sugarcane plant is one of the key waste products that are used in bioethanol production. Therefore, Santiago et al.’s research is fundamental as their identification of expansins can help improve the biomass and yield of the plant to generate bioethanol. Their work might not have been available today had the original dataset not been shared by Riaño-Pachón and Mattiello.
Creating a tool for ribosome profiling
In 2020, Kimchi-Sarfaty et al. published their Data Note ‘Ribosome profiling of HEK293T cells overexpressing codon optimized coagulation factor IX’.
The authors conducted ribosome profiling of two versions of the F9 gene with identical protein amino acid sequences but different nucleotide coding sequences. The profiles of these two variants were not previously available, and Kimchi-Sarfaty et al. shared them openly in their dataset.
François et al. used this dataset to test a new docker package for ribosome profiling called RiboDoc. The authors cited the set’s robust reporting and methods as factors that influenced their choice. “The human dataset was selected because quality controls had been rigorously performed, making it possible for us to compare RiboDoc with data analyzed with different scripts,” noted the authors.
This case study is a great example of how data reuse can facilitate greater scientific discovery and advancement of the field.
Data reuse is a vital part of open data that benefits creators, users, and the wider research community by leading to innovative and impactful research projects. As the open data movement shows no signs of slowing down, we can expect that making research data as reusable as possible will be a prominent part of the future research ecosystem.