How to create a thorough Data Management Plan (DMP)
Funders, governments, and institutions are steadily recognizing their role in encouraging and facilitating data access and sharing. As such, they’re increasingly requesting that their grant holders and researchers develop and implement Data Management and Sharing Plans. In this blog, we outline how to create a plan to help you manage your data, meet funder requirements, and help others access and use your data.
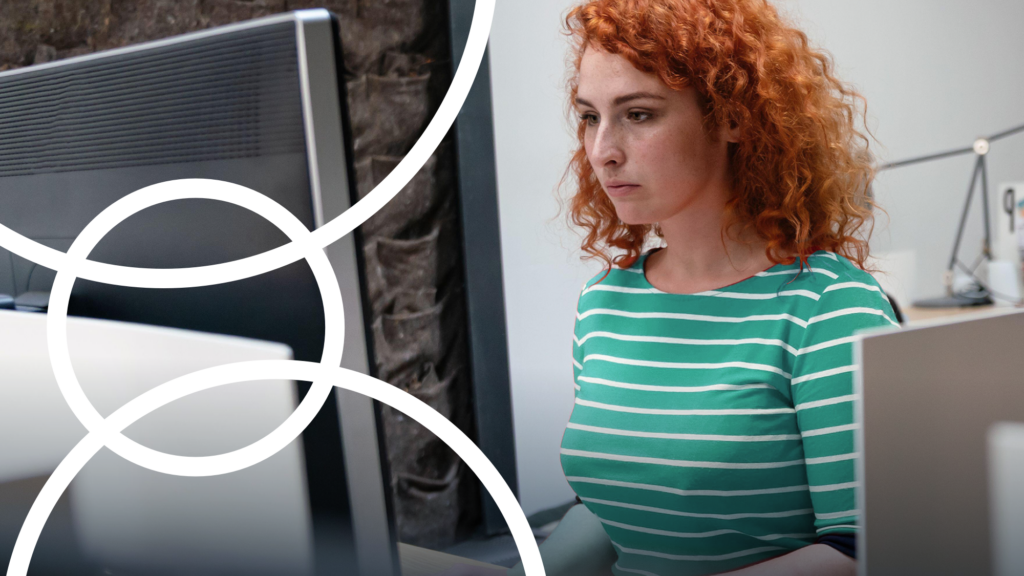
The rise of data sharing and management policies
Globally, research and data sharing policies have grown steadily due to a variety of factors. Events such as the global COVID-19 pandemic highlighted the value of immediate access to research data. Moreover, governments, institutions, and funders are increasingly encouraging their funded researchers to publish their research and data open access (OA).
The turning point
A key turning point has been the implementation of open access policies by national funding bodies like the National Institutes of Health (NIH). As of 2023, NIH-funded researchers are required to publish their research and data OA if the research is funded by the NIH. Additionally, NIH’s Data Management and Sharing Policy, introduced in 2023, requires all scientific data generated by NIH-funded research to be shared with the research community in a timely manner.
What is a Data Management Plan?
In a Data Management Plan, you explain how you intend to use your research data during and after your research project. A Data Management Plan should describe how and what data will be created, outline data sharing and preservation plans, and specify any restrictions that should be applied.
The benefits of creating a Data Management Plan
Having a good data management plan doesn’t have to be mandated by your funder or institution. It has benefits that go beyond simply supporting open data. A Data Management Plan is a living document that changes and grows as your research progresses.
Some of the benefits include:
- Saving time and effort as it forces you to organize your data, prepare it for the next step, and clarify who will have access to it and how.
- Helping you troubleshoot issues with data sharing that need to be resolved before you can share your data effectively.
- Ensuring that you, your collaborators, and other researchers can make use of your data long after your project is over.
Top tips for creating a quality Data Management Plan
The Digital Curation Centre (DCC), a world-leading centre of expertise in digital information curation and building research data management skills, analyzed UK funder’s policies to provide guidance on developing a Data Management Plan.
According to the DCC a thorough plan should cover the following:
#1 Related policies
The first thing you should do is check and define any data policies that are relevant to your research, to understand what information you need to gather and include.
Once you have researched policies, you should list any relevant funder, institutional, departmental, or group policies on data management, sharing, and security. Some of the information you give in the remainder of the Data Management Plan will be determined by the content of other policies. If that is the case, you should link to them in the document too.
#2 Data collection
A thorough Data Management Plan will provide a brief description of the data, including any existing data or third-party sources that will be used. You should also note the data content, type and coverage. Next, you’ll need to outline and justify your choice of format and consider the implications of data format and data volumes in terms of storage, backup and access.
Additionally, you should outline how the data will be collected/created and which community data standards will be used, if applicable. You’ll want to consider how the data will be organised during the project, and mention naming conventions, version control, and folder structures. You should also explain how the consistency and quality of data collection will be controlled and documented.
When it comes to data collection and analysis, there may be discipline-specific (and repository-specific!) guidelines you need to comply with to ensure your data is Findable, Accessible, Interoperable, and Reusable (FAIR). Make sure you’ve done your research and have a clear understanding of best practice in your field.
#3 Ethical implications
Ethical issues affect how you store data, who can access and use it, and how long it is stored. You should describe how you plan on managing ethical concerns, whether that be through data anonymization or formal consent agreements, for instance.
You should also show that you are aware of any issues and have planned accordingly. If you are carrying out research involving human participants, you must also ensure that consent is requested to allow data to be shared and reused.
#4 Legal considerations
You should be prepared to state who will own the copyright and Intellectual Property Rights (IPR) of any data that you will collect or create, along with the licence(s) for its use and reuse. For multi-partner projects, IPR ownership may be worth covering in a consortium agreement. Consider any relevant funder, institutional, departmental or group policies on copyright or IPR. Also consider permissions to reuse third-party data and any restrictions needed on data sharing.
#5 Documentation and metadata
Consider what information is needed for the data to be read and interpreted in the future. Once you have done this, you should explain how the data will be documented and what metadata will accompany the data. This allows other researchers to have sufficient information to understand the source, strengths, weaknesses, and analytical limitations of the data so that they can make informed decisions when using it.
Examples of documentation may include items like data dictionaries, codebooks, protocols, logbooks, or lab journals, README files, research logs, analysis syntax, algorithms, and code comments.
#6 Define how the data will be organized
You will need to present a sound data storage and preservation strategy. To do this, you’ll need to state how often the data will be backed up and to which locations, and whether any copies are being made.
If you choose to use a third-party service, you should ensure that this does not conflict with any funder, institutional, departmental or group policies, for example in terms of the legal jurisdiction in which data are held or the protection of sensitive data.
#7 Data preservation
Describe how data quality will be assured by explaining how the data may be reused. Will it be replicated with a view of validating your research findings, conducting new studies, or for teaching?
Decide which data to keep and for how long. This could be based on any obligations to retain certain data, the potential reuse value, what is economically viable to keep, and any additional effort required to prepare the data for data sharing and preservation. Remember to consider any additional effort required to prepare the data for sharing and preservation, such as changing file formats. Additionally, consider how datasets that have long-term value will be preserved and curated beyond the research lifecycle.
#8 Data sharing
Describe how your research data will be disseminated. Consider where, how, and to whom data with acknowledged long-term value should be made available. The methods used to share data will be dependent on a variety of factors including, the type, size, complexity, and sensitivity of data. If possible, mention earlier examples to show a track record of effective data sharing.
Furthermore, outline any expected difficulties in sharing data with acknowledged long-term value, along with causes and possible measures to overcome these. Restrictions may be due to confidentiality, lack of consent agreements, or IPR, for example. Consider whether a non-disclosure agreement would give sufficient protection for confidential data.
#9 Roles and responsibilities
You should outline the roles and responsibilities for all activities including, data capture, metadata production, data quality, storage and backup, data archiving, and data sharing. Consider who will be responsible for ensuring relevant policies will be respected and name individuals where possible.
Furthermore, carefully consider any resources needed to deliver the plan. This includes, software, hardware, technical expertise, etc. Where dedicated resources are needed, these should be outlined and justified.
#10 Prepare a realistic budget
This isn’t included in the DCC guidance but is another important detail that should be included in your Data Management Plan. Some funder data sharing policies request researchers include the costs associated with data sharing in their plan.
Open data can’t be an afterthought. It’s essential to know at the outset of your research project if you’ll be making your data open, so that you can plan accordingly. Creating a detailed Data Management Plan (DMP) at the start of your project and keeping it updated throughout as a living document will help you stay organized and prepared.