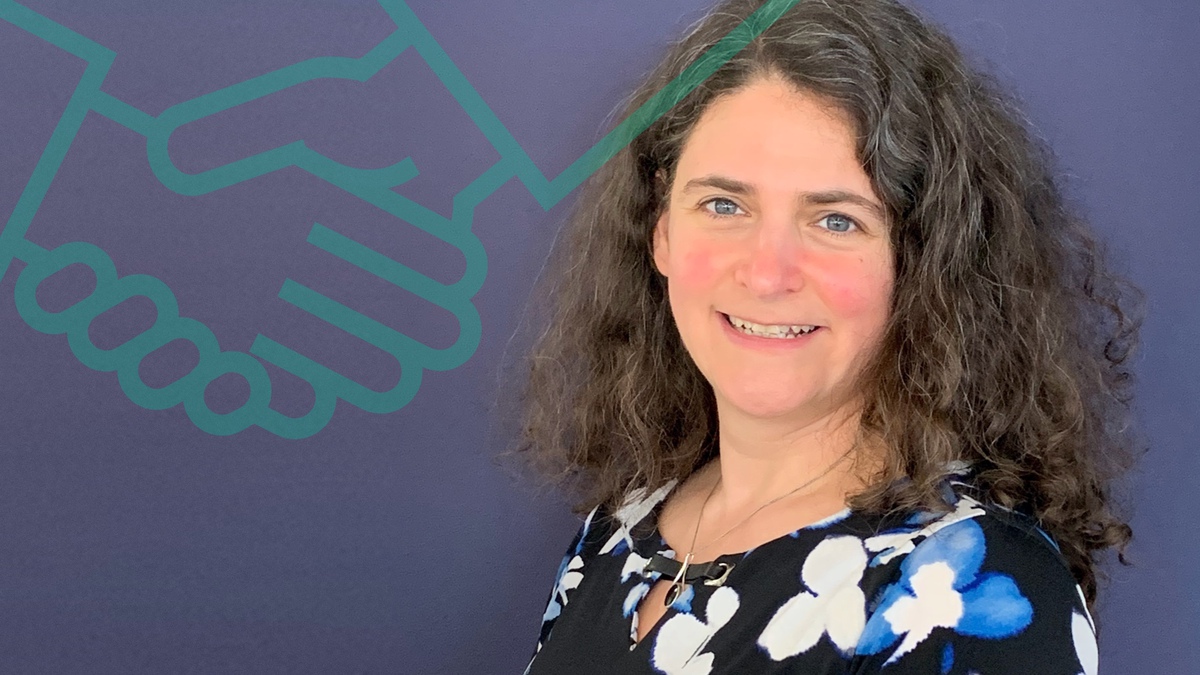
Automatic for the People: how ARWs and open research can work together for public good
Rebecca Lawrence was the academic publisher representative on the USA’s National Academy of Sciences, Engineering and Medicine committee exploring Automated Research Workflows (ARWs). The committee’s recently published report concludes that “the research enterprise stands at an important inflection point” for automation and acceleration, where collaboration, interoperability, and data sharing are critical. Rebecca, as a publisher and scientist who has championed open science and open research for many years, believes that for ARWs to be optimally effective and deliver for public good, we need robust, open platforms and processes, and adequate long-term funding of the infrastructures required to accomplish this. In this blog, she explains why this matters for science and society.
Automated Research Workflows (ARWs) are research processes that integrate cyberinfrastructure, laboratory automation and artificial intelligence (AI) to aid and expedite research, from designing experiments to crunching the data, through to learning from the results to inform further experiments, observations and simulations. The use of ARWs in research has been steadily increasing but their potential to truly accelerate discovery at a much greater order of magnitude has not yet been fully realized.
Machine learning (ML) and AI are transforming the speed and accuracy with which we can interrogate and use scientific data for the public good. The need to expedite research is critical in so many fields, from pandemic epidemiology, climate science and wildfire detection, through to drug discovery, materials science and digital humanities. In particular, Covid-19 illustrated how important it was to rapidly find new treatments by being able to accelerate the time-consuming development, manufacturing and screening of novel antiviral medications. The sudden spread of monkeypox shows again how disease does not respect borders and illustrates the importance of accelerating the research cycle in order to understand and control this and other diseases.
ARWs have real potential to not only greatly improve the speed of research, but also its integrity, reproducibility, replicability and dissemination, but these opportunities also bring challenges. Critical to the success of ARWs is effective FAIR data sharing on which these workflows can be built, but this requires additional tasks and responsibilities of the researchers involved, such as creating, documenting, storing, and sharing scientific software and databases. Furthermore, the quality and accuracy of this data is especially crucial so that small errors or simple bugs in the analysis are not then greatly amplified by the scaling of the automated workflows. ARWs can also benefit dissemination through greater adherence to minimum reporting requirements but to achieve this will require greater agreement on reporting standards across many fields.
In addition, biases in analyses and in algorithms need to be carefully monitored so that self-perpetuation of such biases doesn’t lead to suggested correlations that make no sense. Spotting such biases in closed algorithms though brings new challenges, especially for peer reviewers who are critically assessing new findings at publication. Tackling this challenge will require further exploration and the need to bring in new types of experts as reviewers to provide the adequate level of checks required to know whether to trust that the research conclusions are valid and can be safely built upon. Without this safety net at peer review, we run the risk of ‘cod-science’, with bad data and problematic analyses and conclusions that can then be used to feed conspiracy theories.
At the same time, current publishing and peer review systems are in danger of being overwhelmed by ARWs’ greater volume of research outputs if we don’t start to think smartly now. We need to think again about what really warrants peer review and what types of expertise are best placed to provide the type of review required for different types of outputs. The use of AI in informing further experiments also opens up interesting questions about authorship and the different types of contributions to new research (including the balance between the discipline expert and the workflow developer) and who takes credit for the work if automated workflows are generating their own research questions.
To drive a greater uptake of ARWs and the benefits that they can bring is going to require significant long-term investment in infrastructure and suitable incentives that ensure researchers are adequately rewarded for the task of creating and curating high-quality AI-ready data resources on which ARWs are so reliant. Furthermore, those same incentives that encourage open research behaviours that put an emphasis on reliability rather than just innovation, that reward the sharing of a much broader range of outputs beyond standard research articles, and that value transparency and reproducibility across all phases of the research cycle (including at publication) are going to be essential components for the successful expansion of the use of ARWs in future research. This will require continued progress in shifting research culture, and the development and implementation of new educational programs and career pathways to ensure the research community have the appropriate knowledge and expertise required to successfully implement and utilise ARWs and mitigate against the associated challenges.
Confidence alone in open science and ARWs to bring game-changing benefits to society is not enough. As research communities, governments and research-based industries increasingly recognise the potential of ARWs, much work and collaboration is now needed between these communities and other key partners such as academic publishers, research infrastructures and more to develop the necessary policies, infrastructures, incentives and ultimately funding to support the growth of ARWs to achieve the full potential of this revolution in scientific methodology and its consequent impact on the speed of knowledge progression.
Leave a Reply
Your email address will not be published. Required fields are marked *